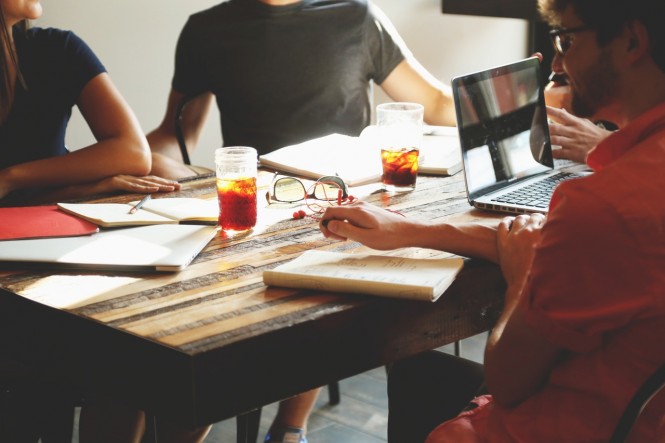
The techniques in this article use a NLP technique called the Meta model which can be used to identify ambiguity and where information is missing.
For an introduction to what NLP is please read the introduction in the following article – How NLP can be used to improve communication with stakeholders
The Meta model was originally presented in a book called The structure of Magic I: A book about language and therapy in 1975 by Richard Bandler and John Grinder.
The language patterns identified and behaviour still apply in requirements gathering and providing guidance on how to recognise when certain behaviours occur and what questions to ask to obtain further information. It is also useful for reviewing requirements documents because when you spot certain words it can be a good indication of where information is missing.
According to the model there are 3 types of language patterns to look out for. These are:
1. Generalisation
2. Deletion of information
3. Distortion
People will always have a more complete internal representation of what they wish to communicate than what they can put into words. Therefore they will shorten the description. As a result they will generalise information, delete most of the material or distort it.
People will generalise. Our minds compare and generalise old similar material with new data allowing us to learn quickly so we do not have to relearn old concepts.
People have to delete some of the information they give. Every second 2 million pieces of information feed into the brain, therefore it makes sense that we have to screen out much of this information.
People will distort to simplify the description of their experience.
The impact this has on the business analyst is that the person giving the requirements may have missed out important information, distorted the information and given generalisations without being specific. The same might apply to you without realising when writing a business requirements document.
To summarise the Meta model is a technique to explore areas of communication that are susceptible to ambiguity.
If we don’t try to remove ambiguity then the requirements gathered will be subject to many different interpretations especially as it gets handed over to different teams such as development and testing. The end result could then be different to the stakeholder’s expectations.
See link to Removing Ambiguity from requirements using NLP for practical examples of how the meta model can be applied in practice.
Thoughts? Questions? Please share in the comments.